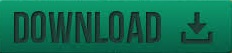
Similarly, volume ETA(4) is another eta that changes only with every site and is associated by.
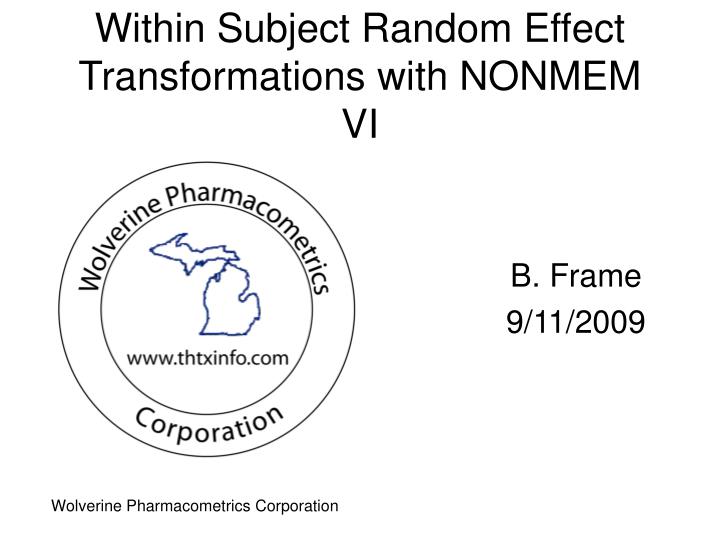
They associate together because they provide random effects to the same individual parameter. We conclude that, except for the situation of modeling standard deviations with only sparse information, NONMEM produces tests of significance that are effective at detecting clinically significant differences between two populations.īy White DB, Cindy Walawander, Liu, D.Y., Thaddeus Grasela. NONMEM is informed that ETA(3) is a CL ETA that changes only with every site and is associated by nesting with CL ETA(1), which varies with each subject. As an example, the nonnormality of estimates of the ratio of standard deviations plays an important role in explaining the low power for the confidence interval tests. These results were then compared to results on parameter estimation in order to gain insight into the question of power. For comparison of the standard deviations, and when the volume of information available was relatively small, however, the likelihood ratio test was more able to detect differences between the two groups.
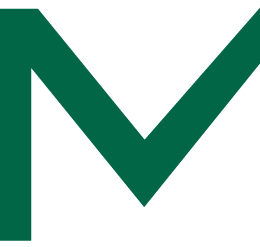
For tests comparing the means, the confidence interval tests had approximately the same power as the likelihood ratio tests and were consistently more faithful to the nominal level of significance. To generate the power curves, a range of these parameters was employed other pharmacokinetic parameters were selected to reflect the variability typically present in a Phase II clinical trial.
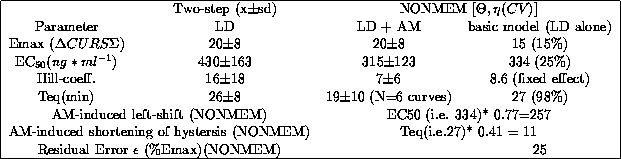
Data were simulated according to a monoexponential model and, in that context, power curves for each test were generated for (i) the ratio of mean clearance and (ii) the ratio of the population standard deviations of clearance. These two methods are the test based upon 95% confidence intervals and the likelihood ratio test. In a simulation study of inference on population pharmacokinetic parameters, two methods of performing tests of hypotheses comparing two populations using NONMEM were evaluated.
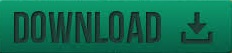